Title | Equality of opportunity in travel behavior prediction with deep neural networks and discrete choice models |
Publication Type | Thesis |
Year of Publication | 2021 |
Authors | Yunhan Zheng |
Academic Department | Department of Urban Studies and Planning and Department of Civil and Environmental Engineering |
Degree | Master in City Planning and Master of Science in Transportation |
University | MASSACHUSETTS INSTITUTE OF TECHNOLOGY |
Abstract | Although researchers increasingly adopt machine learning to model travel behavior, they pre- dominantly focus on prediction accuracy, while largely ignore the ethical challenges and the adverse social impacts embedded in the machine learning algorithms. This study introduces the important missing dimension - computational fairness - to travel behavioral analysis. It highlights the accuracy-fairness tradeoff instead of the single dimensional focus on pre- diction accuracy in the contexts of deep neural network (DNN) and discrete choice models (DCM). The author firstly operationalizes computational fairness by equality of opportunity, then differentiates between the bias inherent in data and the bias introduced by modeling. The models inheriting the inherent biases can risk perpetuating the existing inequality in the data structure, and the biases in modeling can further exacerbate it. The author then demonstrates the prediction disparities in travel behavioral modeling using the National Household Travel Survey 2017. Empirically, DNN and DCM reveal consistent prediction disparities across multiple social groups, although DNN can outperform DCM in prediction disparities because of DNN’s smaller misspecification error. To mitigate prediction dispari- ties, this study introduces an absolute correlation regularization method, which is evaluated with the synthetic and the real-world data. The results demonstrate the prevalence of pre- diction disparity in travel behavior modeling, which can exacerbate social inequity if the prediction results without fairness adjustment are used for transportation policy making. As such, the author advocates for careful considerations of the fairness problem in travel behavior modeling, and the use of bias mitigation algorithms for fair transport decisions. |
URL | https://mobility.mit.edu/sites/default/files/Yunhan_s_Thesis.pdf |
image:
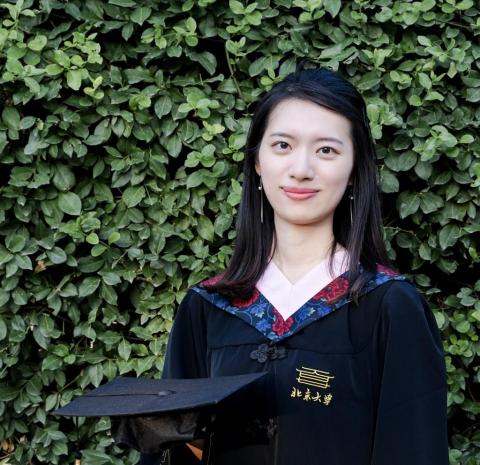